In an era defined by unprecedented data generation, the ability to extract meaningful insights and drive informed decisions has become a critical skill across industries. This surge in data has fueled an explosive demand for data scientists in the USA, professionals equipped with the analytical, statistical, and programming prowess to navigate complex datasets. For individuals looking to enter this burgeoning field or upskill their existing capabilities, enrolling in a comprehensive data science course in the USA is a pivotal step.
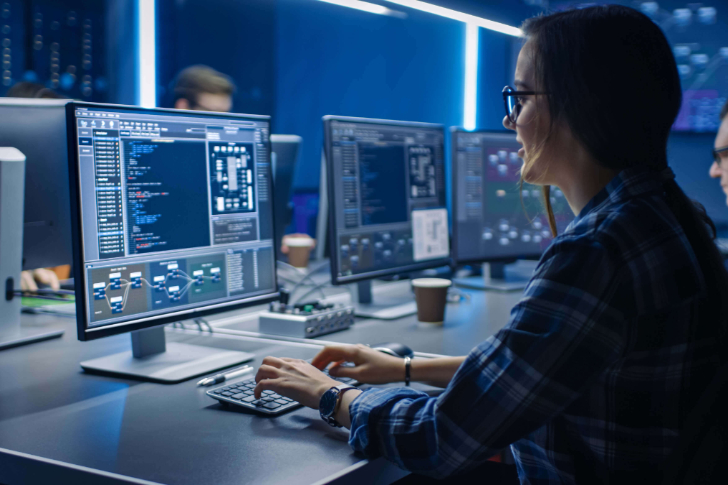
However, the landscape of available programs is vast and varied, ranging from intensive bootcamps to university-backed master’s degrees and flexible online certifications. This article delves into the key considerations for choosing the right data science course in the USA, exploring the different formats, crucial curriculum components, and providing illustrative cost tables to guide your educational investment.
The Educational Spectrum: Exploring Data Science Course Formats in the USA
Aspiring data scientists in the USA have access to a diverse range of educational pathways, each with its own structure, duration, and intensity:
- University Bachelor’s and Master’s Degrees: Traditional university programs offer a comprehensive and theoretical foundation in data science, often encompassing mathematics, statistics, computer science, and specialized data science methodologies. Bachelor’s degrees provide a strong entry point, while master’s degrees offer deeper specialization and advanced skills, often preferred for research-oriented roles or career advancement. These programs typically span several years.
- Data Science Bootcamps: These intensive, short-term programs (typically lasting a few weeks to several months) are designed to rapidly equip individuals with practical, job-ready data science skills. Bootcamps often focus on hands-on projects and real-world applications, making them attractive for career changers or those seeking a fast-tracked entry into the field.
- Online Data Science Courses and Certifications: The rise of online learning platforms has made data science education more accessible and flexible. Numerous platforms offer individual courses, specialized certifications, and even online master’s degrees in data science. These options cater to various learning styles and schedules, allowing individuals to learn at their own pace.
- Professional Development Workshops and Training: These shorter, focused programs are often geared towards professionals looking to acquire specific data science skills or tools relevant to their current roles. They may cover topics like specific programming languages, data visualization techniques, or machine learning algorithms.
Navigating the Curriculum: Essential Components of a Data Science Course
Regardless of the format, a robust data science course in the USA should cover several fundamental areas to equip graduates with the necessary skills:
Communication and Storytelling: The ability to effectively communicate technical findings to both technical and non-technical audiences is crucial for a data scientist’s impact.
Programming Fundamentals: Proficiency in programming languages like Python and R is essential for data manipulation, analysis, and model building. Courses should cover core programming concepts, data structures, and relevant libraries (e.g., NumPy, Pandas, Scikit-learn in Python; dplyr, ggplot2 in R).
Statistical Analysis and Probability: A strong understanding of statistical concepts, probability theory, and hypothesis testing is crucial for interpreting data, drawing inferences, and building reliable models.
Machine Learning (ML): This core area involves learning various algorithms for predictive modeling, classification, clustering, and dimensionality reduction. Courses should cover both theoretical foundations and practical implementation of ML techniques.
Data Wrangling and Preprocessing: Real-world data is often messy and requires cleaning, transforming, and preparing for analysis. Courses should teach techniques for handling missing values, outliers, and data inconsistencies.
Data Visualization: The ability to effectively communicate data insights through compelling visualizations is a critical skill. Courses should cover various visualization tools and techniques (e.g., Matplotlib, Seaborn in Python; ggplot2 in R; Tableau).
Big Data Technologies: As data volumes continue to grow, familiarity with big data tools and frameworks like Spark and Hadoop may be necessary for certain roles.
Database Management and SQL: Understanding database systems and the ability to query and manipulate data using SQL is essential for accessing and working with large datasets.
Domain Knowledge (Optional but Beneficial): Some specialized data science courses may incorporate domain-specific knowledge relevant to industries like healthcare, finance, or marketing.
Ethics and Responsible Data Science: Increasingly important is the understanding of ethical considerations in data collection, analysis, and model deployment, including bias detection and fairness.